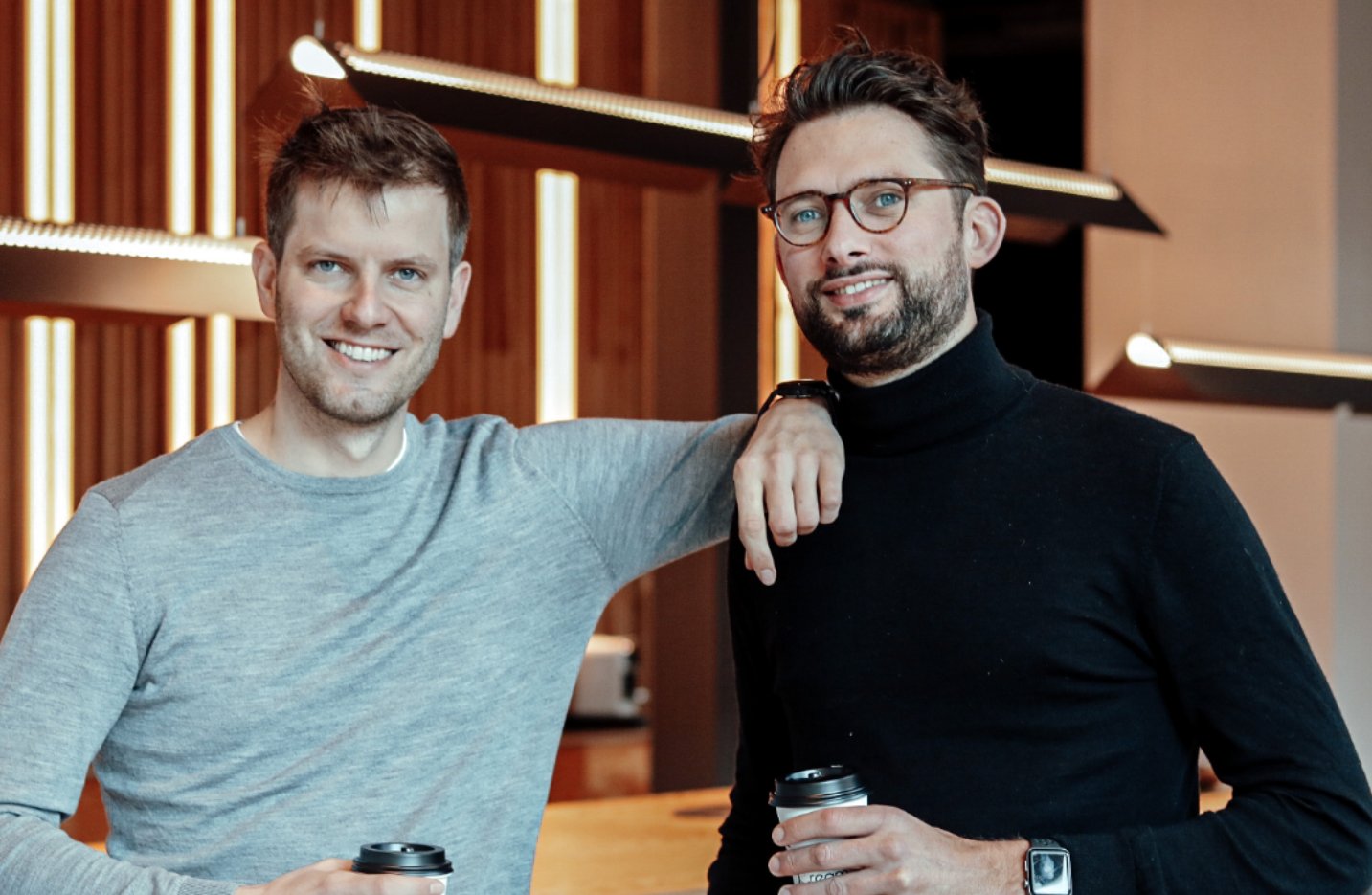
Companies realize value out of data all the time. Yet Gartner estimates that 85% of AI projects still fail, why? The focus in projects is often on getting models in production, however getting it in production is not all that is required. Models need to be incorporated into decision making to actually deliver value, or in other words, they need to be adopted.
At Borg, we help companies increase their success rate of AI projects by managing the non-technical challenges in getting from production to value.
In this article we share 5 common challenges we see in going from production to adoption, and tips on how your ML team can overcome them.
1. Models in production are not used
We have seen many examples within companies where models have been developed and dashboards have been made only to have no one looking at them. From the Data Science team perspective, they have done everything right. Their model is treated as production software. It has a structured release process, predictions exposed through a well documented API and so on. From a total company perspective however, no value was added until the models are used. It is in the adoption step, where the value is generated.
Value is the search engine team that loads improved search suggestions in the index to improve conversion. It is the sales agent reaching out to predicted leads and recording the feedback. It is the customer service department optimizing their staff planning based on predicted call loads. So how come so often models are built but not used?
The reason is that ML teams do not necessarily have the skills or resources to lead the adoption phase after their model is in production. We had a case where a Finance department wanted to use their data to improve their quarterly sales forecasts. We discovered that their central data science team had already built a predictive sales forecasting model. Unfortunately though, it wasn’t used. Building the model and putting it in production was not all that was needed to realize value. It takes project management, domain knowledge on financial control and change management skills to incorporate the forecast in business planning. ML teams should ensure a “translator” role is included in projects that lead the adoption of predictions.
2. Analytics projects take forever to complete
As we have seen, it is in adoption where the value is generated. We have seen many ML teams work primarily on very risky / complex projects requested by the business that sounded great, but have a large chance of failure. As a result, projects can take forever to complete and never seem to reach the desired results.
We see two major drivers of complexity. First the dependencies you have on IT and other stakeholders to reach adoption. Second, the amount of change management required in working with the predictions.
In one of our projects we developed a simple new search suggestion algorithm. However, to get the suggestions to deploy in production, and have an AB measure conversion, we were dependent on four different IT teams. What initially looked like building a straightforward algorithm improvement turned out to require getting on the backlogs of multiple IT teams which were already fully occupied.
ML teams should invest time upfront to figure out what the dependencies on other teams there are for a certain use case. It is not to say that projects that require multiple other teams can’t be done, but you should be well aware of this added complexity. When taking on a use case that requires multiple teams, be sure to have them committed upfront to prevent getting stuck in endless projects.
3. ML teams are swamped in requests
Machine Learning teams are often overloaded with requests and find it difficult to assess the value of all these requests. Not surprisingly, as it would require them to deeply understand the business impact in the department’s they interact with which is not their area of expertise. Machine Learning teams that lack a clear long-term strategy risk working on projects that do not align with the overall strategy of the company, or produce little value.
It also leads to a bias to projects that render results on the short run. We would advise data science teams to also clearly identify the value they can unravel long-term.
Determining what the long-term value drivers can be for a particular data science team requires translating the company’s overall strategy to a specific data strategy with big hairy goals. This should be done in close cooperation with business stakeholders and can be a fun process, for instance by holding ideation workshops with inspiring examples from cutting edge Tech companies. By setting an AI strategy and developing a strategic roadmap, data science teams can focus their efforts on the long-term projects that matter most, in a mix of short-term wins and big bets.
4. End-users are involved (too) late
We see many projects that take way too long before end users are actively working with the predictions. We think that working with predictions is a vital step for three reasons. Having end-users work with the predictions greatly improves the chance of it being used. Secondly, it can reduce throughput time by preventing a “waterfall” approach by first working on model performance. Lastly, the feedback from real-life helps scope engineering efforts
Many will recognize the meeting in which model performance is presented in graphs to end-users and asking them “is this good enough to use?” Surprisingly it never is. To find out what performance is required you need to actually be working with the predictions.
We worked on a project where after 9 months, first predictions were presented to the end-users to work with. In working with the predictions, we learned that the invested months of engineering work to build and train a Machine Learning model had been a waste. A simple ranking algorithm would have sufficed. If end users would have been involved early on, the time to market would have been way faster and the costs of development a lot lower.
We suggest setting up a feedback loop with end users fast. It is also vital to ensure that predictions land in the place where end-users can easily use it. For instance, a model that creates leads for call agents should be integrated into their CRM system. If this cannot be done through available API’s, alternative solutions like robotics process automation could provide the answer.
5. Goal of projects are unclear
We find that the goals, scope and metrics of analytics projects are often not well formulated. Failing to do so reduces focus and will lead to unnecessary delays in projects.
We see that ML teams will work on the predictions and focus on historical model performance. If you do not zoom out and define measures for overall project success, you run the risk of being stuck in model development for a long time. You want to clearly define what the predictions should improve, and how you can measure this.
For example, in fraud detection, you want to prove historical performance of the model. But you also want to measure how well a model is adopted in real life. How many fraud cases were presented to the risk department, how many were investigated and how many were classified as correct / incorrect? As you can quickly see, this ties together our previous points. End users have to work with the predictions. Measuring their adoption of the predictions, and the effective outcome they realize is just as important as measuring the quality of the predictions themselves.
Be sure to critically ask what the goal of a certain project is and don’t be afraid to stop projects if goals are too vague.
Thank you for reading our article on some of the challenges that you might face when conducting analytics projects. We are excited to talk more on these subjects, so if you are interested to learn more, feel free to reach out!
On behalf of Borg Consulting: Eric Prinsen and Eric van der Knaap.